Is there a connection of quantum gravity, Schrödinger's cat and new quantum concepts of machine learning approaches?
In the quantum gravity approach the spacetime becomes a dynamical object, which satisfy some similar relation compared to the well-known uncertainty relation in quantum mechanics. There is an analogy to the position and momentum in quantum mechanics in quantum gravity. These canonical variables are the parallel transport along closed curves and the fluxes through surfaces, which are derived objects from the curvature of the spacetime manifold. The extension of the formalism to parallel transport along curves and fluxes through surfaces is achieved by a spin-network approach. Moreover, the energy quatum is replaced by the fact that area and volume is discretized in the quantum gravity approach.
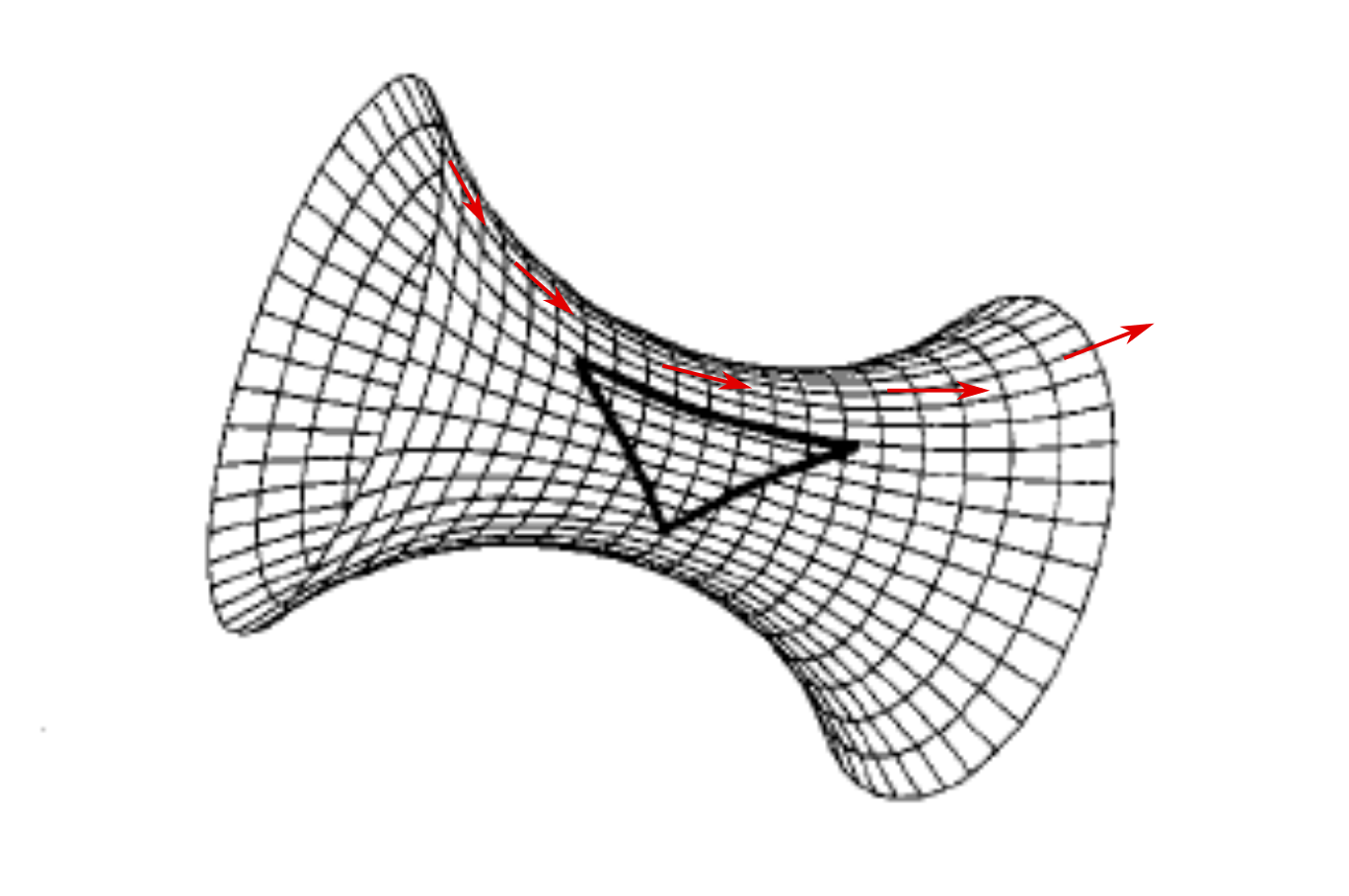
The Schrödingers cat paradox describes the state of a quantum system to be non-classical in the sense that it is a probability of the cat being dead or alive. Only due to the opening of the box (the measurement) the cat (the quantum object) obtains its status (an eigen state of the object). The probability in this experiment can be interpreted as reasonable expectation of the cat representing a state of knowledge (the cat is either alive or dead) and hence can be understood to be a Bayesian probability. After the measurement the knowledge is changed, since the status is afterwards established.In future I am convinced this approach can be applied in machine learning in then field of multi-body-simulations and measurements of complex systems. The idea is to make a broadened use of physical knowledge of the system. The advantage of such an approach is that a smaller amount of data is necessary.
Another important aspect of quantisation is the non-uniform distribution of electromagentic energy of the thermal radiation of a black body in dependence of the frequency of the radiation. The key characteristic of the energy quantum is that it takes only integer multiple values. This idea can be transferred also to neural networks. In the case of an optimization of a large number of weigths in different layers unexpected numerical errors might occur. The weigths can be mapped to integer values by using scaling factors. Then the neural network is supposed to be robust under small changes of the weigths ("noise") such that only the essential pattern is recovered by the trained network. Another advantage of such a mapping method to integers is the reduction of memory and the requirements on computing capacity.
Ph.D. studies in natural science with focus
on general relativity (GR), quantum mechanics, algebraic quantum field theory and quantum gravity:
One of the most interesting research arreas in physics is to find an unifying theory of general relativity and quantum physics. One approach is the so-called Loop Quantum Gravity (LQG),which is based on the quantization of geometric objects. Inspired by the duality of Schrödinger's wave function and the Heisenberg matrix representation formulation, the intenion of the thesis is to derive the algebraic operator formulation of loop quantum gravity approach. It is discussed whether other states (i.e. thermal states) than the Ashtekar-Lewandowski state exists. Such thermal KMS-states appear naturally in algebraic quantum field theory approach.
Current work at Schaeffler Technologies AG & Co.KG
Simulations of dynamical multi-body systems
I am currently working on the dynamical simulations of timing and accessory drives of combustion and hybrid engines and in particular of the mechanical and hydraulic components. My focus lies on mathematical modeling of physical processes and complex assemblies by differential equations, i.e. chain and belt system drive vibrations, contact and friction modeling between parts of an assembly, influence of finite element structures on assembly system resonances and hydraulic valves in tensioning systems. There are quite interesting mathematical phemomena occuring for such non-linear systems i.e. due to contact mechanisms or by coupling of hydraulic and mechanical properties of a system. The development process of simulation model starts usually with the derivation of the equation of motions. To validate the model the stability of the non-linear system and after the numeric implementation of the model also the convergence of the integrator are quite important issues. Finally typical parameter sensitivities are tested (i.e. dependency of mass / moment of inertia).
Often the component is set up independently and then the mathematical algorithm is implemented in an excisting multi-body system simulation in an industrial simulation software. Therefore usually user routines are programmed in different languages i.e. Fortran 90 or C++, which can be compiled for different mbs-software. Moreover it is useful to test different simplified models in Matlab to achieve a robust implementation. For the programming performance it is convenient to use the object oriented language and for the tracebility a version control i.e. by git.
A development process of a simulation model of a component subdivide into several tasks:
a) mathemaical description of the system, integration into an existing system model and validation of the model
b) The verification / functionality test of the component model with respect to expected physical effects i.e. by analytical estimations and
c) the dynamical system behavior of the full system in which the component is integrated.
d) The next step is the validation of the component and full system model compared to measurements. For this purpose different tests are used, i.e. for the estimation of the volume flow of a fluid through a valve, periodical pulsing tests for hydraulic tensioning assembly systems to obtain tensioner hysteresis or engine tests to observe the full tensioning behavior in a drive system. An important part of the work is the statistical data analysis of the measurements for the fixation of the objectiv properties of the component. Indeed it is necessary to exclude the particular influences of the test environment from the measuremnts, since they might overlap the component behavior. Therefore it is important to understand the specific test and to critically evaluate the measurement data. An investigation of the test data without such validation might lead to puzzling conclusions. Furthermore due to complex component and system interaction, effects that are not present at the measurement of the component might appear at full system tests. Thus estimations of the interactions are helpful to interpret the measurement data.
The rapid development of new concepts for machine learning and by an increasing amount of available packages i.e. Tensorflow, Keras, Numpy and Scikit
in Python interesting new methods are available for simulation and measurment data. The training of neural networks with data might lead to new insights and expand the classical optimization and DoE-analysis approaches.
Hobbies
Playing around with modern gadgets i.e. Raspberry Pi and doing a lot of sport in nature!
Ph.D. studies
Diploma thesis 2006:
Teaching experiences:
International conferences:
Interesting lectures:
Important scientific communities:
|